This Apple Self-Driving Tech Could Make Better Cars And AR
Apple research into how self-driving cars could better and more affordably identify pedestrians and other potential hazards has been spotted online, giving a new glimpse into the Cupertino firm's secretive project. The company has long been rumored to be working on autonomous driving technology, with the most recent belief being that it hopes to develop, not an Apple-branded car, but software that it can license out to automakers.
However, while Apple execs have made general comments about the complexity of driverless vehicles, little detail has been shared about what exactly the company hopes to do in the segment. The submission of a new paper on 3D object detection by Apple researchers, however, has lifted the curtain somewhat.
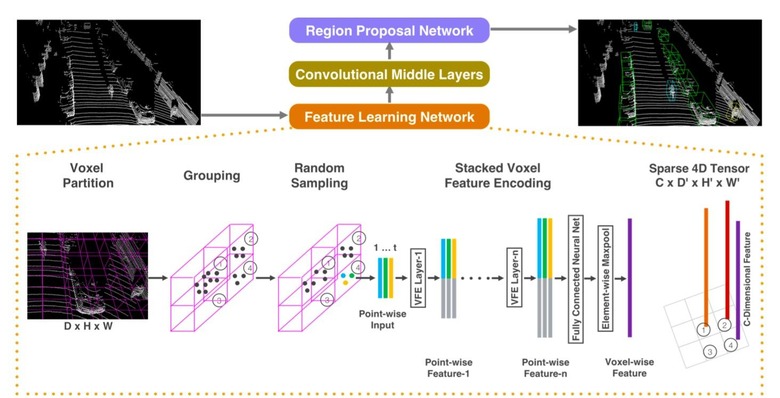
Yin Zhou and Oncel Tuzel are AI researchers at Apple, according to their LinkedIn profiles. They submitted a paper titled "VoxelNet: End-to-End Learning for Point Cloud Based 3D Object Detection" to academic site arXiv on November 17. It describes a method by which the challenge of identifying objects from a 3D point cloud create by a LIDAR scanner could be made more efficient.
LIDAR – or Light Detection and Ranging – sensors are commonplace on autonomous vehicles. They use a fast-spinning laser array which bounces light off targets around the car. By measuring the reflected light, they can build up a so-called point cloud of dots, showing what is present in 3D space.
The scanners themselves are historically very expensive, though efforts from manufacturers in recent years have helped bring those costs down, not to mention slim the hardware itself from the bulky bucket-like protrusions atop older driverless prototypes. Even so, a point cloud in itself isn't enough for a vehicle to navigate the world. From that, the software needs to figure out what each cluster of points represents, whether that is another car, a pedestrian, a cyclist, or something else, and if that presents a possible risk.
The system Zhou and Tuzel outline is dubbed VoxelNet, a 3D detection network that brings together feature extraction and bounding box prediction, and combines it into "a single stage, end-to-end trainable deep network." In effect, it splits the point cloud into chunks of 3D voxels, then transforms a group of points within that chunk into "a unified feature representation" courtesy of a voxel feature encoding (VFE) layer.
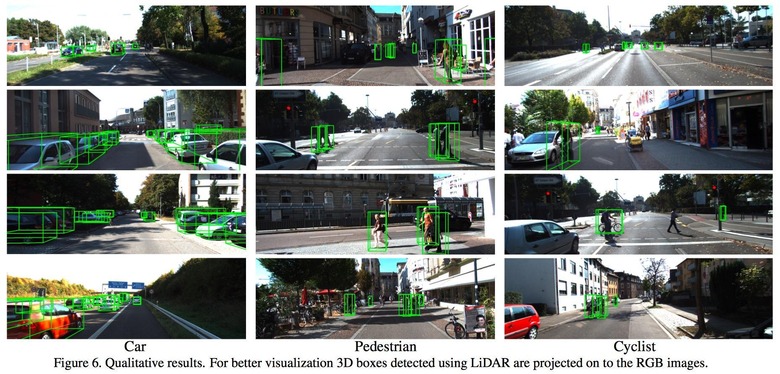
That's handed over to a region proposal network, or RPN, which is encoded with patterns that can be recognized within each chunk. Traditionally, autonomous vehicles would use an image- or video-based RPN, combined with LIDAR, requiring more sensors. However, this new VoxelNet approach allows RPN to be applied directly to a LIDAR point cloud alone.
The result, the researchers write, is that their system can outperform other current LIDAR 3D detection methods "by a large margin" and, in particular, has "highly encouraging" abilities to spot cyclists and pedestrians. That might mean vehicles outfitted with fewer sensors, which would reduce complexity as well as cost.
MORE Apple's autonomous car tech got caught on camera
Although autonomous cars are one possible route for the VoxelNet technology to take, it's not the only one. Zhou and Tuzel suggest that it could be just as applicable to robots and augmented or virtual reality, all of which require a fast and efficient way to identify objects in real-time.
Apple's approach to research has generally been a clandestine one. The company has traditionally been loathe to discuss what it's working on internally until there are products using that technology to show for it. However, while preserving the launch surprise, that strategy has arguably proved counterproductive in acquiring new talent, since scientists and engineers generally want to publicize or at least publicly discuss their work.
As a result, Apple launched the Apple Machine Learning Journal, effectively a blog of select research from its teams. Most recently, it described efforts but are computer vision machine learning team to do face detection via neural networks, but relying solely on on-device processing, rather than uploading data to the cloud as other systems do. Previous research posts have covered handwriting recognition, how Siri detects the "Hey Siri" prompt, and how neural networks themselves can be more efficiently trained.