NYU teaches machines to learn like humans
Scientists at New York University work with machines that'll soon learn like humans, dramatically shortening the time it takes for a robot to adapt. Creativity is what they're teaching, and the robot apocalypse is nearly upon us. Brenden Lake, the paper's lead author and Moore-Sloan Data Science Fellow at New York University, suggests that "this work points to promising methods to narrow the gap for other machine learning tasks."Machine Learning you've likely heard of before. Even if you haven't, you likely use it every day. When you search for something in Google, Google takes what you've searched for and enters it in to its memory of what you've searched for in the past. Google's search machine learns your behavior and proceed to give you results accordingly.
A machine can learn. This part isn't new.
What's new is the way machines learn.
"It has been very difficult to build machines that require as little data as humans when learning a new concept," said Ruslan Salakhutdinov, assistant professor of Computer Science at the University of Toronto. "Replicating these abilities is an exciting area of research connecting machine learning, statistics, computer vision, and cognitive science."
Salakhutdinov is one of three authors of the paper published this week on the subject. Also on the team was Joshua Tenenbaum, MIT professor in the Department of Brain and Cognitive Sciences and the Center for Brains, Minds and Machines.
Above and below you'll see examples of generative works demonstrating the learning abilities of this new program (Mr. learning computer, we'll call him), straight from the original research paper.
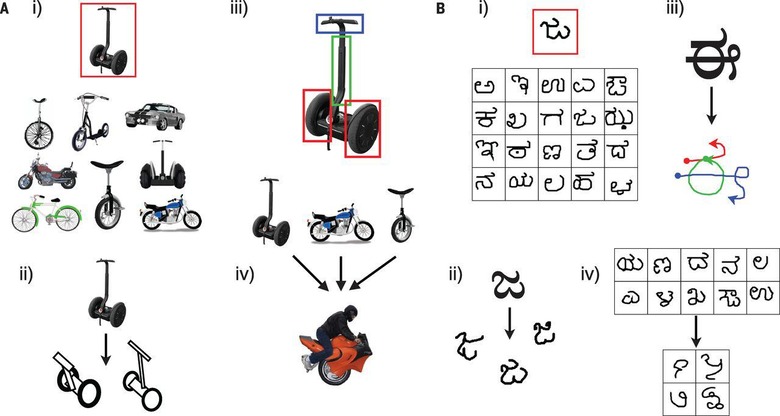
This new work utilizes what they call a "Bayesian Program Learning" (BPL) framework. The program is able to take an example of a concept – a word, for example – and write its own code that's able to then generate examples of different ways in which that word is written. The program can then produce different versions of the word based on what it already understands about different ways in which letters are formed.
"Before they get to kindergarten, children learn to recognize new concepts from just a single example, and can even imagine new examples they haven't seen," said Tenenbaum.
"I've wanted to build models of these remarkable abilities since my own doctoral work in the late nineties. We are still far from building machines as smart as a human child, but this is the first time we have had a machine able to learn and use a large class of real-world concepts — even simple visual concepts such as handwritten characters — in ways that are hard to tell apart from humans."
You can find out more about this subject through Eurekalert and the paper "" as published this week by the scientific journal Science by the authors mentioned above under code DOI: 10.1126/science.aab3050 as of this week.