ReSkin aims to give AI a sense of touch
Humans take the sense of touch for granted, and it's one of the most important types of input that we get in certain situations. Our sense of touch allows us to do things like feel how we are gripping an object to enable us to pick it up easily without crushing it. Anytime we pick up something fragile, like an egg, we know exactly how hard to squeeze it to keep from crushing it, making a huge mess. For robots and AI without a similar sense of touch, it's difficult for the robot to gather information about the object it is attempting to handle.
Facebook AI is working on a new project called ReSkin that is designed to give AI devices additional input. Today, AI can incorporate senses, including vision and sound, but gaining a sense of touch is something that has remained elusive. Part of the reason for limited touch information for AI systems and robots today is limited access to tactile-sensing data.
Researchers working with AI want to incorporate touched data into AI models, but it's very difficult to give AI systems the same sort of touch sensing capability that people have. Facebook AI's ReSkin project is an open-source touch-sensing "skin" created in collaboration between Meta AI researchers and scientists from Carnegie Mellon University. ReSkin helps researchers advance tactile sensing capability in their AI systems quickly and at scale via advanced machine learning and magnetic sensing.
The system is inexpensive, versatile, durable, and replaceable, making it ideally suited for long-term use. ReSkin uses a self-supervised learning algorithm to auto-calibrate the sensor and to make the data generalizable so it can be shared between different sensors and systems. Facebook AI will release the design, documentation, code, and base models to allow researchers everywhere to utilize ReSkin without training with their own data sets. That will allow the integration of tactile sensing into various AI systems quickly.
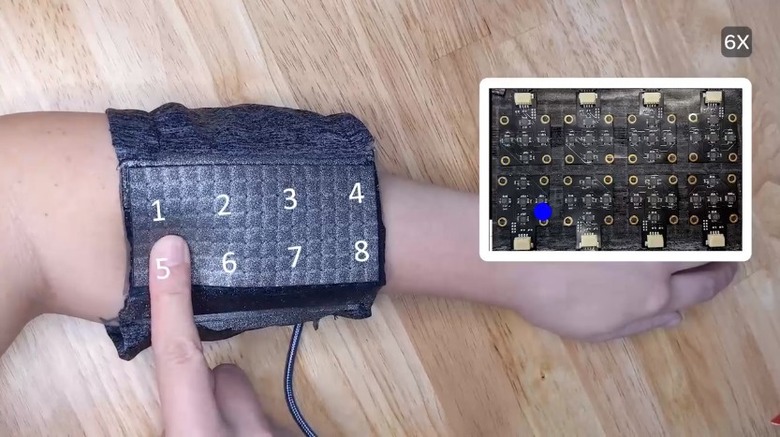
In addition, generalized tactile sensing will allow researchers to collect data that could help advance AI utilized in a range of touch-based tasks. It will be helpful in object classification, proprioception, and robotic grasping. Once AI systems are trained with tactile sensing, they will be capable of new tasks, including work in healthcare settings and the ability to manipulate small, soft, and sensitive objects. The ability to manipulate a wider range of objects could make the robots better suited for packing orders within factories or very sensitive products, including produce.
ReSkin costs less than six dollars to build in 100 unit lots and is even cheaper when produced in larger quantities. The sensor itself is 2 to 3 millimeters thick and can survive 50,000 interactions. Once it wears out, it's easy to replace and offers a high temporal resolution of up to 400 hertz along with a spatial resolution of one millimeter with 90 percent accuracy.
ReSkin also delivers researchers high-frequency three-axis tactile signals allowing systems to adjust during fast manipulation tasks like throwing, slipping, catching, and clapping. The sensor itself utilizes a deformable elastomer that has magnetic particles embedded within it. The elastomer deforms in any direction allowing the magnetic signal to change as it deforms. The changes in the magnetic signal can be measured with a magnetometer allowing information like contact location and the amount of force applied to be translated. Researchers created a generalizable skin to eliminate the need to train a new skin each time the system is replaced.
However, each sensor does go through an initial thorough calibration routine to determine its individual response. The calibration routine is adaptable to tolerate changing properties of soft materials over time. Researchers overcame challenges with ReSkin by removing the need for an electrical connection between the soft material and measurement electronics relying on proximity for the magnetic signals so the electronics only need to be nearby.
Instead of relying on data from a single sensor, the model mapping function utilizes data from multiple sensors. That allowed researchers to train the model on a higher diversity of data, so it produces more generalized and effective data. Instead of collecting calibration data on every new sensor, the self-supervised learning system is used to fine-tune sensors automatically utilizing small amounts of unlabeled data. Facebook AI says work on ReSkin is a component of its commitment to advancing tactile sensing in the AI research domain.